Machine Learning Model Validation for Prediction of Potential PICU Transfer
journals.lww.com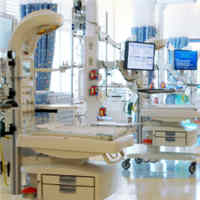
We developed and externally validated a novel machine learning model that identifies ICU transfers in hospitalized children more accurately than current tools.
Our model enables early detection of children at risk for deterioration, thereby creating opportunities for intervention and improvement in outcomes.
Our primary outcome was direct ward to ICU transfer. Using age, vital signs, and laboratory results, we derived logistic regression with regularization, restricted cubic spline regression, random forest, and gradient boosted machine learning models.
Among 50,830 admissions at site 1 and 88,970 admissions at site 2, 1,993 (3.92%) and 2,317 (2.60%) experienced the primary outcome, respectively.
Site 1 data were split longitudinally into derivation (2009–2017) and validation (2018–2019), whereas site 2 constituted the external test cohort.