Clustering Algorithms Usage in Critical Care Research Unravel Patient Heterogeneity
link.springer.com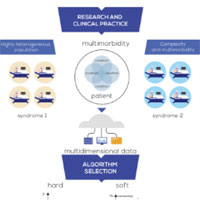
Critically ill patients constitute the most heterogeneous population in the hospital, with the highest rates of acute and chronic multi-morbidity. Daily, two critically ill patients are admitted to the ICU with the same syndrome-based diagnosis, receive similar treatment, and yet have diametrically opposite outcomes.
Increasingly flexible and sophisticated clustering techniques are available, which can allow for analyses of higher-dimensional data-sets that help better characterize patients, disease mechanisms, and heterogeneous treatment response patterns.
Before these findings can truly inform the design of multi-center, international prospective studies and trials, efforts to increase the interpretability of the findings are essential. For instance, the Interpretable Clustering via Optimal Trees algorithm developed by Bertsimas et al. provides a clear, tree-based representation of the most important variables and the respective thresholds which led to cluster formation.