ARDS Subphenotypes Validation and Utility Identified by Machine-learning Models
thelancet.com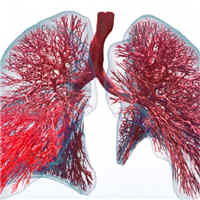
Classifier models using clinical variables alone can accurately assign ARDS subphenotypes in observational cohorts. Application of these models can provide valuable prognostic information and could inform management strategies for personalised treatment, including application of PEEP, once prospectively validated.
In this observational, multicohort, retrospective study, we validated two machine-learning clinical classifier models for assigning ARDS subphenotypes in two observational cohorts of patients with ARDS: Early Assessment of Renal and Lung Injury and Validating Acute Lung Injury Markers for Diagnosis, with LCA-derived subphenotypes as the gold standard.
The primary clinical classifier model had an area under receiver operating characteristic curve (AUC) of 0·92 in EARLI and 0·88 in VALID.
Performance of the primary model was similar when using exclusively EHR-derived predictors compared with manually curated predictors.