Benchmarking Saliency Methods for Chest X-ray Interpretation
medrxiv.org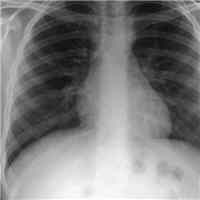
Saliency methods, which “explain” deep neural networks by producing heat maps that highlight the areas of the medical image that influence model prediction, are often presented to clinicians as an aid in diagnostic decision-making.
Although many saliency methods have been proposed for medical imaging interpretation, rigorous investigation of the accuracy and reliability of these strategies is necessary before they are integrated into the clinical setting.
In this work, we quantitatively evaluate seven saliency methods-including Grad-CAM, Grad-CAM++, and Integrated Gradients- across multiple neural network architectures using two evaluation metrics.
We establish the first human benchmark for chest X-ray segmentation in a multilabel classification set up, and examine under what clinical conditions saliency maps might be more prone to failure in localizing important pathologies compared to a human expert benchmark.